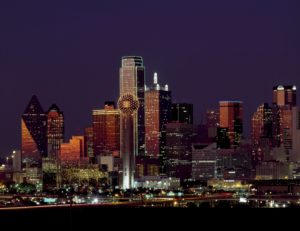
ACMG Meeting 2022
The FDNA team is gearing up for ACMG meeting 2022 and we’re excited to share with you new developments and…
By Dr. Karen Gripp, Chief Medical Officer at FDNA
Next-generation phenotyping (NGP) is characterized by the use of computer learning tools to assess a patient’s presentation (phenotype). For precision medicine to reach its full potential, it requires the combination of NGP with the large data sets derived from next-generation sequencing (NGS).
Before new technologies can be incorporated into the workflow of clinical diagnostic laboratories, validation of results in that setting is necessary. In our presentation at the upcoming ACMG meeting (March 20th, 5:15 pm CT), we will report on the results of such validation studies in different laboratories.
Improved analysis of NGS data through the application of NGP tools was demonstrated in a research context in the “Prioritization of Exome Data by Image Analysis” (PEDIA) study as published by Hsieh et al., Genetics in Medicine, 2019. In this study of cases previously solved through exome analysis, the incorporation of the facial features through Face2Gene, FDNA’s flagship NGP tool, allowed improved ranking of the disease-causing gene. These results suggest that incorporation of NGP tools in the workflow of clinical diagnostic laboratories will increase diagnostic efficiency through automated inclusion of relevant phenotypic data.
Face2Gene LABS was used in the PEDIA approach at the Greenwood Genetic Center on a series of cases submitted for exome analysis. A retrospective data analysis on solved cases showed that gene ranking through Face2Gene LABS improves analytic efficiency through prioritization of the disease-causing variant. Most notably, the added benefit from objective facial image analysis was obvious.
Integration in the clinical diagnostic workflow will vary in different laboratories based on the local preferences and regulation. Thus, validation studies have to reflect the lab’s respective structure and workflow. In contrast to Greenwood Genetics Center, which uses automated facial analysis through Face2Gene routinely, the National University of Singapore does not analyze facial features through Face2Gene. Even without the use of the facial analysis, the AI-driven Face2Gene LABS tool efficiently ranked the disease-causing gene for pathogenic or likely pathogenic variants. This validation study was meaningful to the laboratory because it confirms that Face2Gene LABS performs well, even in the absence of facial feature analysis.
We invite you to attend our presentation at ACMG to learn more about this research, and to envision how Face2Gene LABS can improve your clinical diagnostic analysis pipeline!
To learn more about our offerings and opportunities to collaborate, contact us at info@fdna.com
The FDNA team is gearing up for ACMG meeting 2022 and we’re excited to share with you new developments and…
By Dr. Karen Gripp, Chief Medical Officer at FDNA Next-generation phenotyping (NGP) is characterized by the use of computer learning…
Note: This talk was presented at the 2018 ACMG annual meeting. The video can be seen below, or at this link>…